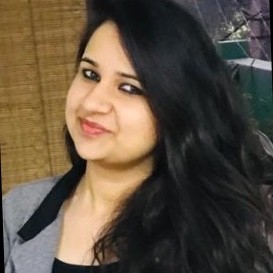
The advent of artificial intelligence (AI) has revolutionized numerous sectors, including the sourcing and recruitment industry. AI-powered tools and platforms promise to streamline the hiring process, improve candidate matching, and enhance overall efficiency. However, with these advancements comes a significant challenge: avoiding bias. AI systems, if not properly managed, can perpetuate or even amplify existing biases, leading to unfair and discriminatory outcomes.
This article explores strategies and best practices to avoid bias when using AI for sourcing.
Understanding AI Bias
Bias in AI can originate from several sources:
- Training Data: AI models are trained on historical data. If this data contains biases, the AI will learn and replicate these biases.
- Algorithm Design: The design of the algorithm itself can introduce bias, especially if it emphasizes certain attributes over others.
- Human Intervention: Human decisions and inputs in the AI development and deployment processes can introduce or exacerbate bias.
Recognizing Bias in AI Sourcing
Bias in AI sourcing can manifest in various forms:
- Gender Bias: AI may favor one gender over another based on historical hiring data that reflect gender disparities.
- Racial Bias: Historical data reflecting racial inequalities can lead to AI models that discriminate against certain racial groups.
- Age Bias: Older candidates may be overlooked if the AI model prioritizes younger, less experienced profiles.
- Educational Bias: AI might unduly favor candidates from certain educational backgrounds or institutions.
Strategies to Avoid Bias in AI Sourcing
1. Diverse and Representative Training Data
The foundation of an unbiased AI system is diverse and representative training data. This involves:
- Collecting Comprehensive Data: Ensure the training data encompasses a wide range of demographics, experiences, and backgrounds. This helps the AI to learn patterns that are more reflective of the real-world population.
- Regular Audits: Regularly audit your training data to identify and rectify any imbalances or gaps. This can involve statistical analysis to check for underrepresented groups.
2. Bias Detection and Mitigation Techniques
Implementing techniques to detect and mitigate bias is crucial:
- Bias Detection Algorithms: Use algorithms specifically designed to detect biases in AI models. These tools can help identify unfair patterns and disparities in how the AI processes data.
- Fairness Constraints: Apply fairness constraints during the model training phase to ensure that the AI does not favor one group over another. Techniques like re-weighting or resampling the data can help achieve this balance.
3. Transparent and Explainable AI
Transparency and explainability are key to identifying and correcting bias:
- Explainable AI (XAI): Use XAI techniques to make the decision-making process of the AI transparent. This allows human reviewers to understand how the AI is making its decisions and to identify any biases that may be present.
- Transparency Reports: Publish transparency reports that outline how the AI system works, the data it uses, and the measures taken to prevent bias. This fosters accountability and trust.
4. Human-in-the-Loop Systems
Incorporating human oversight is essential:
- Human Review Panels: Establish review panels that include diverse members to regularly evaluate the AI’s decisions and outputs. This helps catch biases that the AI might overlook.
- Feedback Loops: Create feedback loops where humans can provide input on the AI’s performance and flag any biased decisions. This continuous feedback helps improve the AI over time.
5. Ethical Guidelines and Governance
Strong ethical guidelines and governance frameworks are crucial:
- Ethical AI Frameworks: Develop and adhere to ethical AI frameworks that prioritize fairness, accountability, and transparency. These frameworks should guide all aspects of AI development and deployment.
- Bias Monitoring Committees: Set up committees to monitor and evaluate the AI for bias regularly. These committees should have the authority to make changes to the AI system if biases are detected.
6. Ongoing Training and Education
Education plays a pivotal role in combating bias:
- Bias Awareness Training: Provide training for all personnel involved in AI development and deployment on the risks and impacts of bias. This includes understanding how biases can be introduced and the importance of mitigating them.
- Continuous Learning: Encourage continuous learning and adaptation. AI is an evolving field, and staying updated on the latest techniques and best practices is essential.
Case Studies and Real-World Applications
Case Study 1: Amazon’s AI Recruitment Tool
Amazon developed an AI recruitment tool to streamline hiring processes. However, it was discovered that the tool was biased against women. The AI had been trained on resumes submitted over a decade, which predominantly came from men. This historical bias led the AI to favor male candidates. Amazon eventually scrapped the tool, highlighting the importance of careful data selection and bias monitoring.
Case Study 2: Unilever’s AI Hiring Process
Unilever successfully implemented an AI-driven hiring process that involved digital interviews and game-based assessments. The company took significant steps to ensure the process was fair and unbiased, including using diverse training data and regularly auditing the system for bias. The result was a more efficient hiring process that also improved diversity within the company.
Future Directions
The field of AI sourcing is rapidly evolving, and the future holds promising developments aimed at reducing bias:
- Advanced Fairness Algorithms: Continued research into fairness algorithms that can more effectively detect and mitigate bias.
- Cross-Industry Collaboration: Greater collaboration between industries to share best practices and develop standardized guidelines for bias-free AI sourcing.
- Regulatory Frameworks: The establishment of regulatory frameworks that mandate fairness and transparency in AI applications, ensuring companies adhere to high standards.
Conclusion
Avoiding bias when using AI for sourcing is both a technical and ethical challenge. It requires a multi-faceted approach that includes using diverse training data, implementing bias detection and mitigation techniques, ensuring transparency and explainability, incorporating human oversight, adhering to ethical guidelines, and providing ongoing training and education. By taking these steps, organizations can harness the power of AI to improve their sourcing processes while ensuring fairness and equality. The journey towards unbiased AI is ongoing, but with diligent effort and a commitment to ethical practices, it is achievable.
View Jobs – https://www.shrofile.com/jobs.php
Let Shrofile Executive Search Find You Transformational Talent
Does your company need a dynamic and forward-thinking leader? Get in touch today and learn more about how we find and place transformational talent.
For more Updates
Website – www.shrofile.com
Facebook page – https://www.facebook.com/shrofile/
Twitter page – https://twitter.com/shrofile
Linkedin Page – https://www.linkedin.com/company/shrofile/
YouTube Page – https://www.youtube.com/channel/UChVfJqpTjx1dHJ2xq_dMtAw
Instagram Page – https://www.instagram.com/Shrofile/